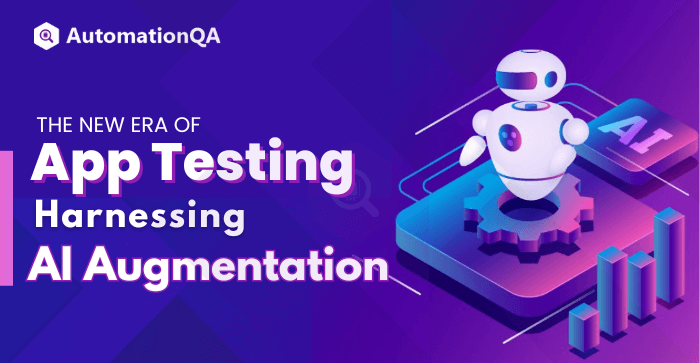
Today, mobile applications have become an integral part of daily lives. From communication to entertainment, these apps have become indispensable tools. Automation companies are, therefore, searching for fresh approaches to quicken the development procedure. It is where AI augmentation testing software provides value by making the testing process easier and more efficient. It automates repetitive tasks to accelerate testing timelines while enhancing the testing coverage.
Due to their increased functionality, modern applications are becoming more complex. Further, companies must ensure a faster release to stay competitive in the market. They cannot sacrifice the caliber of their final product in the process. Therefore, AI has emerged as a game-changer by introducing ML capabilities to the testing process in an era marked by the relentless evolution of application development. So, let’s explore a new era of innovation and optimization in the app testing domain with AI.
What Is AI Augmentation?
In AI-augmented testing, a human tester uses existing AI technologies to improve the testing process. He can use different AI tools for various activities, such as data prediction, test case prioritization, and test data generation. What makes this different from AI testing is the human tester’s involvement.
What AI-Augmentation Testing Can Do
AI-assisted testing is pivotal in enhancing the software testing process’s efficiency, accuracy, and effectiveness. Here are critical aspects of how it transforms software testing.
Automatic Test Case Update
An AI testing tool can continuously monitor applications and track changes, updating test cases accordingly. It reduces the effort required to maintain test scenarios over time, making the testing process more effective and adaptive.
Visual Locators
AI applications utilize visual locators to analyze applications’ visual elements, including user interfaces and graphical components. By scrutinizing screenshots and images, AI enhances testing accuracy and ensures a seamless user experience by monitoring interactions between visual elements.
Test Data Generation
Test data is the soul of the testing process, and AI can breathe life into it. It can help testing automation companies generate diverse data sets to cover exceptional cases. It can quickly produce data that closely matches the actual users.
Self-Healing Tests
AI detects failures or unexpected behavior during test executions and automatically attempts self-healing processes to resolve issues. By adjusting test environments and addressing problems autonomously, AI minimizes disruptions and saves time in the testing process.
Continuous Improvement
The fact that AI improves with each use may be its most significant benefit when it comes to testing. AI learns what worked and what didn’t from test outcomes. Further, the automation testing company can use it to leverage past data and trends to understand patterns and make better recommendations.
Log Analysis
Analyzing logs is a critical step in testing because they can provide great insight into the software’s workings. However, these logs can be very long and complex for humans to decode quickly. AI-assisted testing uses various tools to help humans identify patterns and detect anomalies.
Elastic Scaling Of Testing
AI-based testing solutions efficiently allocate resources, ensuring optimal resource utilization for load, performance, and functional testing. This elasticity enables comprehensive testing coverage while maintaining efficiency and scalability.
AI-Powered Predictive Bots
The test automation company uses AI-powered predictive bots to leverage machine learning algorithms for identifying defects and risks in software. By analyzing historical data, trends, and patterns, these bots proactively predict issues, enhancing the effectiveness of the testing process and improving software quality.
Analytics Of Test Automation Data
AI is critical in analyzing test data by helping testers find trends and patterns. It gives them the necessary insight about the test, essential for making intelligent decisions. AI tools use all the data produced during the test to deliver critical information humans might miss. Thus, AI makes the testing process more efficient and ensures that it becomes more accurate.
Benefits Of Using AI In The Software Testing Process
Automation Of Repetitive Tasks
Automation testing tools with AI capabilities can carry out routine testing procedures and scenarios. It shortens the testing period and lessens the chance of human error. Furthermore, AI-powered intelligent test automation frameworks can rapidly generate, run, and manage test cases with unmatched speed and accuracy, freeing human testers to concentrate on more essential testing tasks.
Predictive Analysis And Anomaly Detection
AI makes predictive analysis and anomaly detection easier, allowing testers to see possible problems before they arise in practical situations. Artificial intelligence (AI) algorithms can detect patterns, trends, and anomalies that point to underlying issues or performance bottlenecks by evaluating large amounts of testing data. By taking a proactive stance, developers of QA automation can address problems early in the development lifecycle, reducing the likelihood of expensive mistakes and delays.
Intelligent Test Case Generation And Optimization
AI-driven methods like reinforcement learning and genetic algorithms make intelligent test case generation and optimization possible. This automation maximizes testing productivity, optimizes resource allocation, reduces the need for manual script design, and ensures comprehensive test coverage.
Continuous Testing And Integration
Artificial Intelligence (AI) facilitates seamless communication between the development and testing teams by enabling continuous testing and integration across the software development lifecycle. Organizations can expedite time-to-market and feedback loops by automating the validation of code changes in real-time by incorporating AI-powered testing tools into continuous integration and delivery pipelines. By utilizing an iterative process, businesses may create high-quality software that fosters agility, creativity, and responsiveness while also meeting the evolving needs and expectations of end users.
How To Implement AI Augmentation In Application Testing
Implementing AI-assisted testing requires a strategic approach to maximize its benefits effectively.
Evaluate The Testing Procedures
Assess your current testing processes, tools, and workflows to identify areas where AI-assisted testing might increase productivity, accuracy, and efficacy.
Identify Appropriate Use Cases
Ascertain which particular use cases will benefit most from applying AI-driven testing strategies, such as test case creation, predictive analysis, anomaly detection, and continuous testing.
Invest In AI Infrastructure And Tools
Obtain or create AI-powered frameworks and solutions tailored to your company’s demands. To effectively enable AI-driven testing, invest in a strong AI infrastructure comprising hardware, software, and data management tools.
Collect And Prepare Quality Data
Gather top-notch data sets from past testing cycles, production environments, and user interaction to appropriately train AI systems. Testing automation companies must ensure the data is appropriately cleaned, tagged, and annotated for data to be as helpful as possible for training AI models.
Train And Fine-Tune AI Models
Depending on the type of testing tasks to be performed, AI models can be taught using supervised, unsupervised, or reinforcement learning strategies. AI models are always being adjusted and optimized in response to user feedback and evolving testing requirements.
Integrate AI Into Testing Workflows
Integrate AI-powered tools seamlessly into your current toolchains and workflows for testing. Supplement AI augmentation into pipelines for continuous integration and delivery to facilitate real-time testing and feedback loops.
Ensure Ethical Use Of AI
Give ethical issues, such as data privacy, bias reduction, accountability, and openness, top priority while testing AI. Put governance and safety measures in place to reduce possible hazards and guarantee that regulations are followed.
Provide Training And Support
Invest in testing teams’ upskilling and training so they can use AI-driven tools and methods to their full potential. Offer continuous assistance, materials, and coaching to enable testers to adjust to AI and fully use its advantages.
Collaborate Across Team
To guarantee alignment and synergy in AI-driven testing efforts, encourage cooperation between the automation testing company‘s development, testing, and operations teams. Provide explicit communication lines and workflows to encourage information sharing and cross-functional collaboration.
Measure And Evaluate Performance
Create measurements and key performance indicators (KPIs) to assess how AI-assisted testing affects software testing activities and its effectiveness. Iterate on tactics, continuously evaluate performance against predetermined benchmarks, and make data-driven adjustments as necessary.
Wrap Up!
Artificial intelligence has completely changed conventional testing techniques, bringing a new era of dependability, efficacy, and efficiency in quality assurance. Through the integration of AI-driven automation, testing processes have undergone a profound evolution, significantly enhancing their performance. Fundamental AI-driven techniques such as anomaly detection, natural language processing (NLP), machine learning (ML), and intelligent testing have raised the quality standard within organizations.
By leveraging these advanced technologies, organizations can achieve higher test coverage, streamline test script creation, detect defects early in the development cycle, and maintain tests adaptively. Embracing AI Augmentation ensures the robustness of software products and positions organizations at the forefront of innovation and competitiveness in today’s dynamic digital landscape.
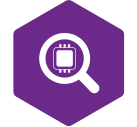
AutomationQA
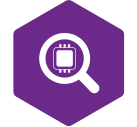